Предыдущая статья — Играем в GTA V c Python. Часть V: алгоритм Хафа.
Сейчас мы начинаем заниматься серьезными вещами. Мы уже определили края дороги, выбрали область интереса (ROI) и в конце концов распознали линии. Наш код на данный момент имеет такой вид:
import numpy as np from PIL import ImageGrab import cv2 import time from directkeys import ReleaseKey, PressKey, W, A, S, D def draw_lines(img, lines): try: for line in lines: coords = line[0] cv2.line(img, (coords[0],coords[1]), (coords[2],coords[3]), [255,255,255], 3) except: pass def roi(img, vertices): mask = np.zeros_like(img) cv2.fillPoly(mask, vertices, 255) masked = cv2.bitwise_and(img, mask) return masked def process_img(original_image): processed_img = cv2.cvtColor(original_image, cv2.COLOR_BGR2GRAY) processed_img = cv2.Canny(processed_img, threshold1=200, threshold2=300) processed_img = cv2.GaussianBlur(processed_img, (3,3), 0 ) vertices = np.array([[10,500],[10,300], [300,200], [500,200], [800,300], [800,500]], np.int32) processed_img = roi(processed_img, [vertices]) # edges lines = cv2.HoughLinesP(processed_img, 1, np.pi/180, 180, np.array([]), 20, 15) draw_lines(processed_img,lines) return processed_img def main(): last_time = time.time() while(True): screen = np.array(ImageGrab.grab(bbox=(0,40, 800, 640))) new_screen = process_img(screen) print('Loop took {} seconds'.format(time.time()-last_time)) last_time = time.time() cv2.imshow('window', new_screen) #cv2.imshow('window2', cv2.cvtColor(screen, cv2.COLOR_BGR2RGB)) if cv2.waitKey(25) & 0xFF == ord('q'): cv2.destroyAllWindows() break
Теперь наша цель — определить по этим линиям настоящую дорожную разметку.
Мы планируем просто вставить свою функцию — и все. Возможно, это не лучший вариант, и мы надеемся, что кто-то придумает что-нибудь еще. Но пока этого не произошло, наш код по крайней мере работает.
Сначала мы находим много линий. Затем, сравнивая их наклон и смещение, находим группы линий, похожих друг на друга, и сохраняем их как «одинаковые линии». Затем берем две наиболее типичные линии и предполагаем, что это и есть наши линии разметки (линии разделения полос движения). После того как мы задали область нашего интереса, следующими наиболее вероятными линиями будут линии разметки. Во всяком случае, такова наша гипотеза.
def draw_lanes(img, lines, color=[0, 255, 255], thickness=3): # if this fails, go with some default line try: # finds the maximum y value for a lane marker # (since we cannot assume the horizon will always be at the same point.) ys = [] for i in lines: for ii in i: ys += [ii[1],ii[3]] min_y = min(ys) max_y = 600 new_lines = [] line_dict = {} for idx,i in enumerate(lines): for xyxy in i: # These four lines: # modified from http://stackoverflow.com/questions/21565994/method-to-return-the-equation-of-a-straight-line-given-two-points # Used to calculate the definition of a line, given two sets of coords. x_coords = (xyxy[0],xyxy[2]) y_coords = (xyxy[1],xyxy[3]) A = vstack([x_coords,ones(len(x_coords))]).T m, b = lstsq(A, y_coords)[0] # Calculating our new, and improved, xs x1 = (min_y-b) / m x2 = (max_y-b) / m line_dict[idx] = [m,b,[int(x1), min_y, int(x2), max_y]] new_lines.append([int(x1), min_y, int(x2), max_y]) final_lanes = {} for idx in line_dict: final_lanes_copy = final_lanes.copy() m = line_dict[idx][0] b = line_dict[idx][1] line = line_dict[idx][2] if len(final_lanes) == 0: final_lanes[m] = [ [m,b,line] ] else: found_copy = False for other_ms in final_lanes_copy: if not found_copy: if abs(other_ms*1.2) > abs(m) > abs(other_ms*0.8): if abs(final_lanes_copy[other_ms][0][1]*1.2) > abs(b) > abs(final_lanes_copy[other_ms][0][1]*0.8): final_lanes[other_ms].append([m,b,line]) found_copy = True break else: final_lanes[m] = [ [m,b,line] ] line_counter = {} for lanes in final_lanes: line_counter[lanes] = len(final_lanes[lanes]) top_lanes = sorted(line_counter.items(), key=lambda item: item[1])[::-1][:2] lane1_id = top_lanes[0][0] lane2_id = top_lanes[1][0] def average_lane(lane_data): x1s = [] y1s = [] x2s = [] y2s = [] for data in lane_data: x1s.append(data[2][0]) y1s.append(data[2][1]) x2s.append(data[2][2]) y2s.append(data[2][3]) return int(mean(x1s)), int(mean(y1s)), int(mean(x2s)), int(mean(y2s)) l1_x1, l1_y1, l1_x2, l1_y2 = average_lane(final_lanes[lane1_id]) l2_x1, l2_y1, l2_x2, l2_y2 = average_lane(final_lanes[lane2_id]) return [l1_x1, l1_y1, l1_x2, l1_y2], [l2_x1, l2_y1, l2_x2, l2_y2] except Exception as e: print(str(e))
Ура! Работает! Полный код на данный момент:
import numpy as np from PIL import ImageGrab import cv2 import time from numpy import ones,vstack from numpy.linalg import lstsq from directkeys import PressKey, W, A, S, D from statistics import mean def roi(img, vertices): #blank mask: mask = np.zeros_like(img) #filling pixels inside the polygon defined by "vertices" with the fill color cv2.fillPoly(mask, vertices, 255) #returning the image only where mask pixels are nonzero masked = cv2.bitwise_and(img, mask) return masked def draw_lanes(img, lines, color=[0, 255, 255], thickness=3): # if this fails, go with some default line try: # finds the maximum y value for a lane marker # (since we cannot assume the horizon will always be at the same point.) ys = [] for i in lines: for ii in i: ys += [ii[1],ii[3]] min_y = min(ys) max_y = 600 new_lines = [] line_dict = {} for idx,i in enumerate(lines): for xyxy in i: # These four lines: # modified from http://stackoverflow.com/questions/21565994/method-to-return-the-equation-of-a-straight-line-given-two-points # Used to calculate the definition of a line, given two sets of coords. x_coords = (xyxy[0],xyxy[2]) y_coords = (xyxy[1],xyxy[3]) A = vstack([x_coords,ones(len(x_coords))]).T m, b = lstsq(A, y_coords)[0] # Calculating our new, and improved, xs x1 = (min_y-b) / m x2 = (max_y-b) / m line_dict[idx] = [m,b,[int(x1), min_y, int(x2), max_y]] new_lines.append([int(x1), min_y, int(x2), max_y]) final_lanes = {} for idx in line_dict: final_lanes_copy = final_lanes.copy() m = line_dict[idx][0] b = line_dict[idx][1] line = line_dict[idx][2] if len(final_lanes) == 0: final_lanes[m] = [ [m,b,line] ] else: found_copy = False for other_ms in final_lanes_copy: if not found_copy: if abs(other_ms*1.2) > abs(m) > abs(other_ms*0.8): if abs(final_lanes_copy[other_ms][0][1]*1.2) > abs(b) > abs(final_lanes_copy[other_ms][0][1]*0.8): final_lanes[other_ms].append([m,b,line]) found_copy = True break else: final_lanes[m] = [ [m,b,line] ] line_counter = {} for lanes in final_lanes: line_counter[lanes] = len(final_lanes[lanes]) top_lanes = sorted(line_counter.items(), key=lambda item: item[1])[::-1][:2] lane1_id = top_lanes[0][0] lane2_id = top_lanes[1][0] def average_lane(lane_data): x1s = [] y1s = [] x2s = [] y2s = [] for data in lane_data: x1s.append(data[2][0]) y1s.append(data[2][1]) x2s.append(data[2][2]) y2s.append(data[2][3]) return int(mean(x1s)), int(mean(y1s)), int(mean(x2s)), int(mean(y2s)) l1_x1, l1_y1, l1_x2, l1_y2 = average_lane(final_lanes[lane1_id]) l2_x1, l2_y1, l2_x2, l2_y2 = average_lane(final_lanes[lane2_id]) return [l1_x1, l1_y1, l1_x2, l1_y2], [l2_x1, l2_y1, l2_x2, l2_y2] except Exception as e: print(str(e)) def process_img(image): original_image = image # convert to gray processed_img = cv2.cvtColor(image, cv2.COLOR_BGR2GRAY) # edge detection processed_img = cv2.Canny(processed_img, threshold1 = 200, threshold2=300) processed_img = cv2.GaussianBlur(processed_img,(5,5),0) vertices = np.array([[10,500],[10,300],[300,200],[500,200],[800,300],[800,500], ], np.int32) processed_img = roi(processed_img, [vertices]) # more info: http://docs.opencv.org/3.0-beta/doc/py_tutorials/py_imgproc/py_houghlines/py_houghlines.html # rho theta thresh min length, max gap: lines = cv2.HoughLinesP(processed_img, 1, np.pi/180, 180, 20, 15) try: l1, l2 = draw_lanes(original_image,lines) cv2.line(original_image, (l1[0], l1[1]), (l1[2], l1[3]), [0,255,0], 30) cv2.line(original_image, (l2[0], l2[1]), (l2[2], l2[3]), [0,255,0], 30) except Exception as e: print(str(e)) pass try: for coords in lines: coords = coords[0] try: cv2.line(processed_img, (coords[0], coords[1]), (coords[2], coords[3]), [255,0,0], 3) except Exception as e: print(str(e)) except Exception as e: pass return processed_img,original_image def main(): last_time = time.time() while True: screen = np.array(ImageGrab.grab(bbox=(0,40,800,640))) print('Frame took {} seconds'.format(time.time()-last_time)) last_time = time.time() new_screen,original_image = process_img(screen) cv2.imshow('window', new_screen) cv2.imshow('window2',cv2.cvtColor(original_image, cv2.COLOR_BGR2RGB)) #cv2.imshow('window',cv2.cvtColor(screen, cv2.COLOR_BGR2RGB)) if cv2.waitKey(25) & 0xFF == ord('q'): cv2.destroyAllWindows() break
from IPython.display import Image Image(filename='lane-finder-example.png')
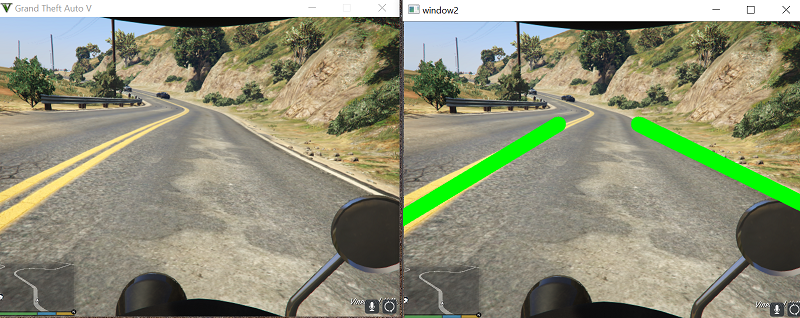
Эти полосы не всегда идеальны. Иногда у нас возникают ситуации, когда обе «внешние линии» образуют только одну полосу движения, что прискорбно. Но мы уже можем начать использовать эти данные для наших целей по обучению искусственного интеллекта. В следующей статье мы рассмотрим один из таких вариантов!
Следующая статья — Играем в GTA V c Python. Часть VII: беспилотный автомобиль.